Trying to understand the stock market without expertise can be confusing and overwhelming. Many people can relate to that feeling! Luckily, this is where Artificial Intelligence can help. It’s not about fancy terms or complicated technology; it’s simply about making things easier and more accessible for everyone involved with numerous applications, such as using AI for pattern recognition in finance.
In recent years, AI has made its way into the financial sector, quietly evolving the way we interact with our wealth, from the way we save to how we invest. But let’s talk numbers for a moment. The growth rate of AI in finance is nothing short of impressive. The global AI in Fintech market, valued at $10.2 billion in 2022, is expected to surge to $46.2 billion by 2028, growing at a CAGR of 28.6% from 2022 to 2028. This isn’t just about the financial institutions benefiting from AI; it’s about making the financial world more navigable for the common user.
As AI evolves, its role in financial decision-making and advisory services has expanded significantly. What started as simple automation for repetitive tasks has now grown into sophisticated systems capable of recognizing patterns, predicting market trends, and offering personalized financial advice.
Here, we’re exploring how AI-driven pattern recognition is simplifying finance. It’s not about big, flashy changes. The primary focus is on the real impact AI has by making complex data easy to use for everyday financial choices. Let’s see how AI is making a real difference in our financial lives.
Understanding AI and Pattern Recognition in Finance
Let’s break it down: AI and Machine Learning (ML) are like the smart assistants of the financial world. They’re here to make our lives simpler by handling the heavy lifting of data analysis. AI and ML learn from data to make smart decisions, and they are getting better and smarter over time by learning and re-learning the algorithms presented.
Now, on to pattern recognition in finance. It’s about spotting the patterns in financial data that might not be obvious at first glance. This could be anything from predicting which way the stock market will swing next week to understanding how people like to spend their money.
So, what kinds of financial data do these smart assistants work with? They dive into everything from market trends, which show us the big picture of what’s happening in the stock market. It even gives us a glance at consumer behavior, providing insight into how people make buying and saving decisions. AI can further process information from sources such as receipts, wishlists, items in our carts, surveys, etc, keeping a thorough track of users’ product preferences.
By understanding and analyzing various types of data, AI and ML in finance help make sense by turning complex information into actionable insights. Just a smart way to make the financial world more accessible to everyone.
The Mechanism of AI in Pattern Recognition
AI can employ various algorithms and models to analyze financial data. These tools enable AI to process vast amounts of information efficiently, identifying patterns and trends that are not immediately obvious. They are as follows:

AI’s Role in Leading Conventional Banks
Here’s a closer look at how some of the leading financial firms are leveraging AI’s pattern recognition capabilities to drive innovation and achieve remarkable results:

At the forefront of integrating AI into financial services, JPMorgan Chase utilizes advanced neural networks for analyzing market trends and optimizing trade strategies. This technology allows the firm to process and interpret complex datasets, identifying patterns that human analysts might overlook. As a result, JPMorgan has seen significant improvements in its trading operations, achieving better trade execution and more effective risk management. Which in turn enhances profitability and reduces exposure to volatile market movements.

Meanwhile, Goldman Sachs has turned to machine learning, particularly decision trees, to refine its credit scoring models. By analyzing a wide array of data, including transactional history and behavioral patterns, the firm can accurately assess the creditworthiness of individuals and businesses. This approach has led to more nuanced risk assessments, reduced default rates, and enabled Goldman Sachs to extend credit more confidently. It further efficiently leverages a broader range of borrowers, fostering greater financial inclusion.
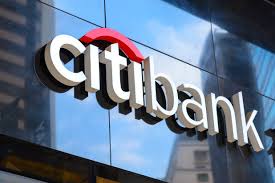
Furthermore, by utilizing clustered algorithms, Citibank has advanced its customer segmentation efforts. It identifies distinct groups within their customer base based on spending habits, preferences, and financial behavior. This granular understanding allows for highly personalized marketing campaigns and service offerings. This significantly enhances customer engagement and satisfaction. The results have been clear: increased customer loyalty, higher conversion rates on marketing initiatives, and a more nuanced understanding of the diverse needs of their clientele.
These examples demonstrate how AI’s pattern recognition capabilities are applied in the financial services industry. By leveraging AI, companies like JPMorgan Chase, Goldman Sachs, and Citibank can make more informed decisions and reduce risks. It further allows them to tailor their services to meet the needs of their customers more effectively.
Applications of AI-driven Pattern Recognition in Finance
The solutions offered by AI-driven pattern recognition are transformative, efficient, and also grounded in data-driven insights. Here’s how this technology is being applied across various facets of finance:
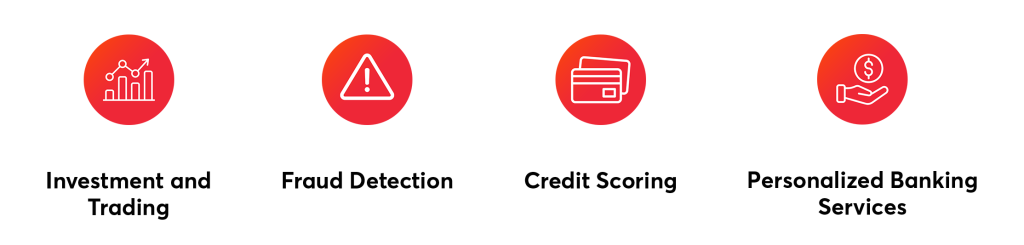
Investment and Trading
AI uses past and current market data to forecast future trends, helping investors make smarter choices. For example, it can pinpoint the right moments to buy or sell stocks by spotting patterns in market behavior. This approach helps reduce risks and improve investment outcomes.
A real-world instance is Renaissance Technologies, a hedge fund that leverages AI to analyze and trade in the stock market. Their AI-driven strategy, particularly with the Medallion Fund, has significantly beaten market averages by accurately predicting stock movements. This success story highlights AI’s effectiveness in simplifying and enhancing investment processes.
Fraud Detection
AI plays a vital role in spotting and terminating fraud by checking transactions for unusual patterns. This method helps prevent fraud before it happens, making financial services safer and building customer trust.
Mastercard offers a clear example of using AI and machine learning for fraud detection. They use AI to monitor transaction patterns, quickly identifying anything suspicious, like a card normally used in New York suddenly buying items in another country. This fast detection reduces fraud risks significantly, saving a lot of money and maintaining secure transactions for users.
Credit Scoring
AI powers credit scoring by evaluating a wide array of data, not just credit history. This includes looking at transaction records, spending patterns, and even social media behavior to judge credit risk. This thorough analysis leads to more accurate credit scores, helping lenders make informed decisions and expanding credit access.
For example, Upstart, a lending platform, uses AI to go beyond traditional credit checks. It considers various factors like education, job history, and where applicants live. This approach has identified reliable borrowers who might not have qualified under old systems, widening loan accessibility and lowering the rate of defaults.
Personalized Banking Services
AI greatly improves banking by personalizing services to fit each customer’s unique spending patterns and financial goals. This leads to tailored advice, products, and services, ensuring that every customer receives what best suits their needs.
Bank of America’s virtual assistant, Erica, exemplifies this by offering personalized banking advice to millions. Erica analyzes transactions to provide custom financial tips, bill reminders, and savings suggestions. For instance, if Erica notices a pattern of overdraft fees for a customer, it might recommend opening a savings account or revising their budget. This level of personalization improves the banking experience, helping customers manage their finances more wisely.
Challenges and Ethical Considerations

What’s Next?
One thing that’s for sure is that the usage of AI for pattern recognition in finance looks promising, with ongoing advancements in machine learning and data analytics paving the way for even more sophisticated applications.
In this blog, we’ve seen how AI is transforming finance by enhancing decision-making, efficiency, and innovation. All this despite facing challenges like data bias and privacy concerns. VentureDive stands at the forefront of all this, offering expert Data and AI solutions tailored for the fintech sector. Discover how we can help navigate these complexities and find new opportunities for your business.